The Ultimate Guide to Machine Learning Labeling Tools
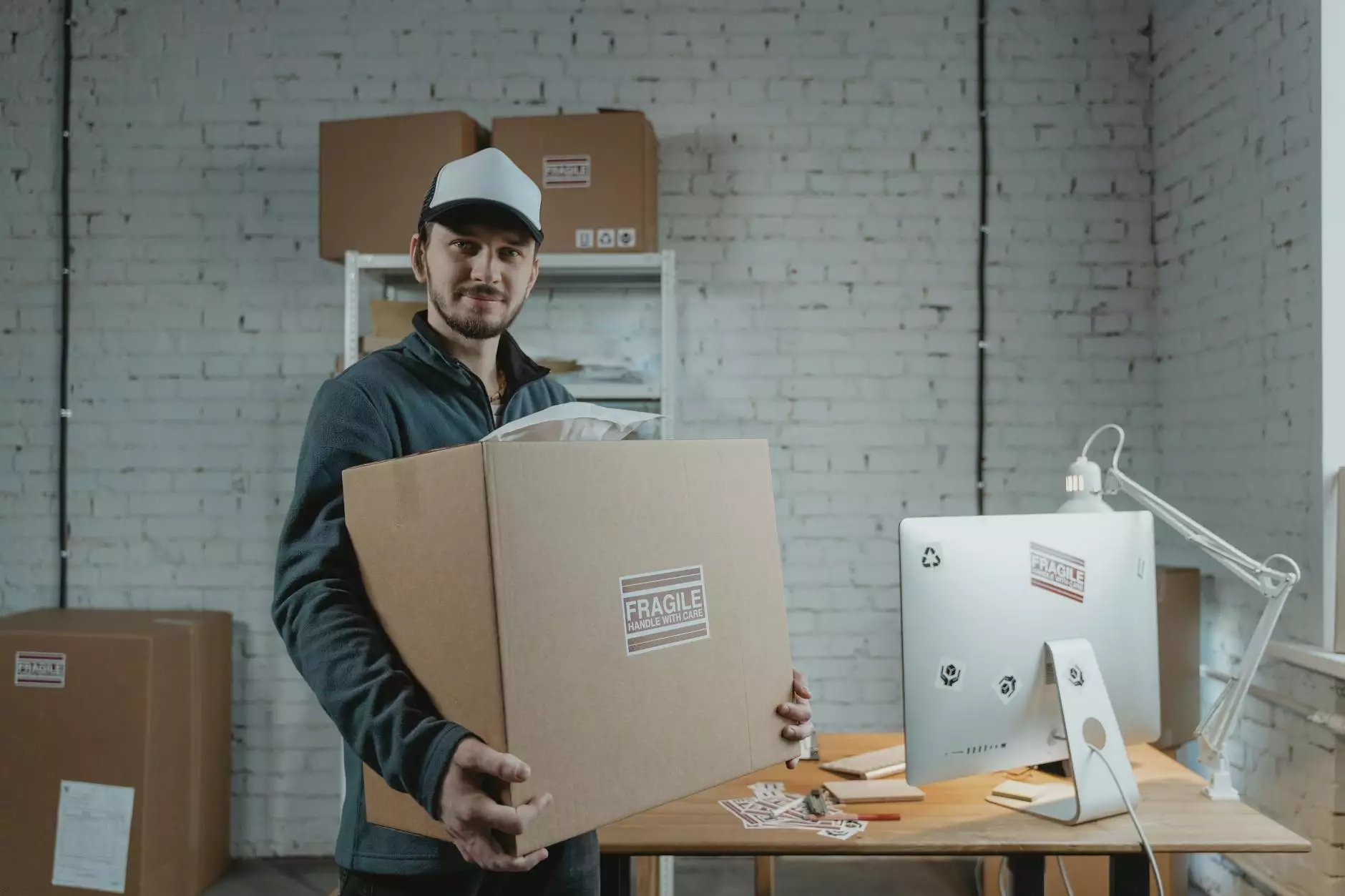
In the rapidly evolving landscape of artificial intelligence (AI) and machine learning (ML), the role of machine learning labeling tools has become pivotal. As industries increasingly rely on AI for insights, decision-making, and automation, the demand for accurate data annotation and labeling has surged. In this comprehensive guide, we will explore what machine learning labeling tools are, their importance, the features to look for, and how Key Labs’ state-of-the-art data annotation platform can elevate your AI projects.
What is a Machine Learning Labeling Tool?
A machine learning labeling tool is a software application designed specifically to assign labels to raw data. These labels help machines understand and learn from data, enabling them to make informed decisions. Labeling is essential for supervised learning, where algorithms learn from input-output pairs, thereby improving their performance over time.
Key functionalities include:
- Automatic Annotation: Some tools use pre-trained models to suggest labels for new data.
- Manual Labeling: Allows human annotators to input labels for data that requires a more nuanced understanding.
- Quality Control: Maintains the accuracy and consistency of the labeling process through various mechanisms.
The Importance of Data Annotation in Machine Learning
Data is the backbone of any machine learning project. However, raw data is often unstructured and not ready for machine learning applications. This is where data annotation comes into play. Here are several reasons why data annotation is vital:
- Enhanced Model Accuracy: Properly labeled data enables ML algorithms to generalize better, leading to improved performance.
- Informed Decision Making: Accurate annotations provide insights that lead to better business outcomes.
- Scalability: Automated labeling tools can accelerate the annotation process for large datasets, making it feasible to tackle various machine learning projects.
Common Types of Data Annotations
When it comes to data annotation, various methods are utilized depending on the data type. Here are some common types:
1. Image Annotation
This includes labeling images for computer vision applications. Common techniques involve:
- Bounding Boxes: Placing rectangular boxes around objects in images.
- Semantic Segmentation: Assigning a label to every pixel in an image.
- Polygonal Annotation: Outlining complex shapes and objects.
2. Text Annotation
Text annotation involves labeling textual data for various applications, including:
- Entity Recognition: Identifying and categorizing entities within the text.
- Sentiment Analysis: Classifying the sentiment expressed in the text.
- Topic Tagging: Assigning labels based on the topic discussed in the text.
3. Audio Annotation
Audio annotation is crucial for speech recognition and other audio processing applications. It often includes:
- Transcription: Converting spoken language into text.
- Speaker Identification: Labeling audio segments by speaker.
- Sound Classification: Identifying different sounds or events in audio recordings.
Choosing the Right Machine Learning Labeling Tool
With so many options available, selecting the right machine learning labeling tool can feel overwhelming. Here are some factors to consider:
1. Usability
The tool should have an intuitive interface that allows users to navigate effortlessly. Ensuring that the annotation process is user-friendly can significantly enhance productivity.
2. Flexibility
Your projects may demand different types of annotations, so it's crucial to choose a tool that supports various data types—be it images, text, or audio.
3. Integration Capabilities
Look for tools that can seamlessly integrate with your existing software stack. This is particularly important for teams that need to maintain workflows across various platforms.
4. Quality Assurance Features
Quality control mechanisms, such as review workflows or consensus scoring, can help ensure that outputs remain consistent and accurate.
5. Scalability
The tool should be able to handle increasing volumes of data as your projects grow. This often means looking for solutions that use machine learning to enhance the annotation process.
Key Labs: A Leader in Data Annotation Tools
Key Labs offers an advanced data annotation platform that encompasses all the essential features for effective data labeling. With a reputation for high-quality outputs and user satisfaction, Key Labs stands out in the crowded market of machine learning labeling tools.
Features of Key Labs' Data Annotation Platform
- Multi-format Support: Works with images, text, audio, and video, so you're covered no matter your data type.
- Intelligent Automation: Use AI-driven suggestions to speed up the annotation process and offer suggestions for manual labeling tasks.
- Customizable Workflows: Tailor annotation processes to match your specific project needs.
- Robust Quality Control: Tools such as double-blind reviews and confidence scoring to maintain accuracy.
- Collaboration Tools: Facilitate team collaboration, allowing multiple users to work on projects simultaneously.
Benefits of Using Key Labs' Machine Learning Labeling Tools
Choosing Key Labs as your partner in data annotation comes with a plethora of advantages:
1. Increased Efficiency
By leveraging intelligent automation and collaborative tools, you can significantly increase the speed and efficiency of your data labeling processes.
2. High Accuracy Rates
The combination of human expertise and AI technology ensures that outputs are not just fast but remarkably accurate, driving better training outcomes for your ML models.
3. Cost-Effective Solutions
Outsourcing annotation tasks can be costly, but with Key Labs, you have a scalable solution designed to fit various budgetary constraints without compromising on quality.
4. Comprehensive Support
Key Labs offers unparalleled customer support, guiding you through the entire annotation process, from setup to execution.
Success Stories with Key Labs
Many companies across various sectors have found success by implementing Key Labs’ data annotation platform. Here are a few noteworthy examples:
1. Healthcare Innovation
A leading healthcare provider utilized Key Labs for annotating thousands of medical images. The precise labeling enabled the development of an AI model that improved diagnostic accuracy and reduced the time needed for image evaluations.
2. Retail Analytics
A major retail chain employed Key Labs for text annotation, labeling customer feedback to identify trends and sentiments. This data drove significant marketing strategies, ultimately increasing customer satisfaction and sales.
3. Autonomous Vehicles
One of the top players in autonomous vehicle technology turned to Key Labs to label vast datasets of video and image feeds. The high-quality data significantly improved the model’s ability to detect and respond to obstacles in real-time.
Conclusion
The role of machine learning labeling tools in AI development cannot be overstated. As businesses race to leverage AI for competitive advantage, understanding the intricacies of data annotation becomes increasingly vital. Key Labs’ robust data annotation platform not only enhances the efficiency of this process but also ensures that your data is of the highest quality.
For those looking to revolutionize their AI projects, investing in the right labeling tools is essential. With Key Labs, you are equipped to face the challenges of the modern data landscape—empowering your organization to make informed decisions and drive innovation.