Leveraging the Power of Labeling Tool Machine Learning for Business Growth
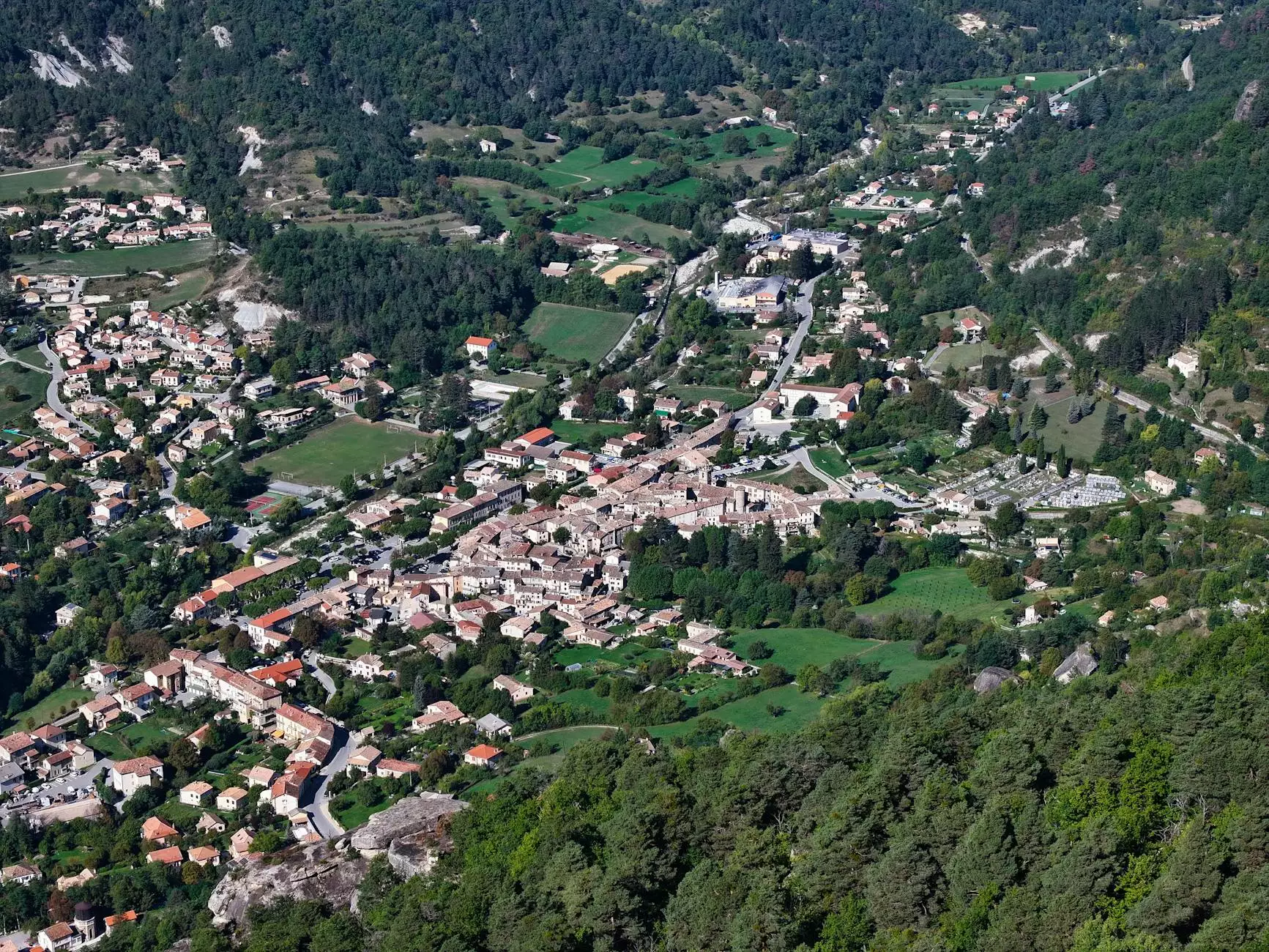
In today’s rapidly evolving digital landscape, businesses across various sectors are leveraging technology to streamline operations, enhance productivity, and gain competitive advantage. One of the most significant innovations in recent years is the advent of labeling tool machine learning, a transformative approach to data annotation that is reshaping how organizations approach software development and data-driven decision-making.
Understanding Labeling Tool Machine Learning
At its core, labeling tool machine learning refers to the application of machine learning algorithms to automate the data labeling process. This technology is essential for training machine learning models, as high-quality labeled data is required for AI systems to learn and make accurate predictions.
Machine learning thrives on data, and data must be correctly labeled to be effective. Manual labeling, while accurate, is often time-consuming and prone to human error. This is where labeling tools powered by machine learning come in, significantly enhancing the efficiency of data annotation and improving the overall quality of datasets used in AI applications.
The Importance of Data Labeling in Machine Learning
Data labeling is a crucial step in the machine learning process, affecting everything from model training to performance evaluation. Here’s why it matters:
- Quality of the Model: The accuracy of machine learning models is directly linked to the quality of the labeled data. High-quality labels lead to better model predictions.
- Speed of Development: Automated labeling speeds up the process, allowing companies to train their models faster and bring products to market more quickly.
- Cost Efficiency: Reducing the need for extensive manual labor translates to lower costs in the long run.
How Labeling Tool Machine Learning Works
The labeling tool machine learning process usually involves several key steps:
1. Data Collection
The first step involves gathering large volumes of raw data, which could range from images and videos to text and audio files. This data serves as the foundation for the machine learning model.
2. Preprocessing Data
Before any labeling can occur, the collected data often requires preprocessing. This stage may involve cleaning the data, handling missing values, or transforming data formats for compatibility with algorithms.
3. Automated Labeling
Using trained models, labeling tools can automatically generate labels for the data. For instance, an image recognition algorithm can identify objects in images and label them accordingly.
4. Human-in-the-Loop Verification
While automation significantly enhances labeling speed, human oversight remains essential. Human reviewers validate and correct these labels to ensure high accuracy, especially in complex scenarios.
5. Continuous Learning
Machine learning models continuously improve over time. As more data is annotated and the models receive feedback, their accuracy and reliability increase, making them more effective.
Benefits of Using Labeling Tool Machine Learning
The integration of labeling tool machine learning within software development processes can yield numerous advantages:
- Accelerated Annotation Process: Automated tools drastically reduce the time required for data annotation, allowing teams to focus on other critical areas of development.
- Improved Accuracy: Machine learning can achieve higher consistency in labeling by minimizing human error and bias, especially when handling large datasets.
- Scalability: As data volumes grow, scaling the annotation process becomes simpler with automated tools that handle larger datasets without proportional increases in cost or time.
- Cost Savings: The reduction in manual labeling resources translates into significant cost savings for organizations.
Applications of Labeling Tool Machine Learning
The applications of labeling tool machine learning span various industries, reflecting its versatility and importance in modern technology. Here are several key areas where this technology is making an impact:
1. Healthcare
In healthcare, accurate data labeling is essential for developing diagnostic tools and treatment planning systems. Machine learning labeling tools assist in labeling medical images, patient records, and clinical notes, thereby improving patient outcomes through enhanced predictive analytics.
2. Autonomous Vehicles
The development of self-driving cars relies heavily on machine learning. Labeling tools are used to annotate images and video from vehicle sensors, ensuring autonomous systems can recognize signs, pedestrians, and other vehicles accurately.
3. Marketing and Customer Insights
In marketing, businesses utilize labeling tools to analyze customer behaviors. By labeling customer feedback, social media interactions, and purchase histories, companies can gain valuable insights into consumer trends and preferences.
4. Natural Language Processing
In natural language processing (NLP), labeled data is crucial for tasks such as sentiment analysis, entity recognition, and language translation. Labeling tools streamline the annotation of textual data to enhance machine learning models that understand human language.
Challenges in Labeling Tool Machine Learning
Despite its advantages, there are challenges associated with implementing labeling tool machine learning, including:
- Quality Assurance: Ensuring the quality of automatically generated labels still requires human intervention, which can become a bottleneck if not managed effectively.
- Domain Expertise: Certain domains may require expert knowledge for accurate labeling, making automation less effective without proper training data.
- Data Privacy Concerns: Handling sensitive data for machine learning purposes raises concerns about privacy and data protection, necessitating strict compliance with regulations.
Choosing the Right Labeling Tool for Your Business
When selecting a labeling tool machine learning solution for your organization, consider the following factors:
- Compatibility: Ensure the tool integrates seamlessly with your existing systems and workflows.
- Scalability: Choose a solution that can grow with your data needs.
- User-Friendly Interface: A user-friendly interface can simplify the labeling process for your team, reducing training time.
- Support and Training: Robust customer support and training options can facilitate smoother implementation and usage.
Future Trends in Labeling Tool Machine Learning
The future of labeling tool machine learning is incredibly promising. As technology continues to evolve, we can expect several emerging trends:
- Increased Use of Deep Learning: Deep learning techniques will continue to enhance the accuracy and capabilities of labeling tools.
- AI-Assisted Labeling: AI will play a pivotal role in creating more intelligent labeling systems that can learn from user feedback and improve over time.
- Greater Collaboration Across Industries: Cross-industry partnerships will lead to the development of more comprehensive datasets and improved labeling tools adapted for specific use cases.
- Regulatory Framework Development: As data privacy concerns grow, a clearer regulatory framework will emerge, guiding the ethical use of labeling technologies.
Conclusion: The Impact of Labeling Tool Machine Learning on Business
In conclusion, the adoption of labeling tool machine learning represents a significant advancement in the field of software development and data science. By automating the data labeling process, businesses can enhance their operational efficiency, improve the accuracy of their AI models, and make data-driven decisions with greater confidence.
As organizations continue to embrace this technology, the potential for innovation and growth is substantial. The combination of high-quality labeled data with sophisticated machine learning algorithms is paving the way for breakthroughs across industries, ultimately transforming the way businesses operate and compete in the digital age.
To explore more about how labeling tool machine learning can benefit your business and enhance your software development processes, visit keymakr.com.